Case Study: The Art of the Constraint
Introduction
The Indiana Artificial Intelligence (AI) Innovation Network brings together stakeholders from across industries and verticals to provide those working in the AI space with the support and connections they need to keep Indiana at the forefront of technology. With more than 150 members, the AI Innovation Network meets monthly with in-person and virtual options. Meetings feature content from Indiana’s AI innovators, networking opportunities, and more. Case studies are published quarterly to highlight the wide array of business-focused topics around AI innovation.
In Q1, 2025, AI Innovation Network member Data317 shared two groundbreaking projects that use AI to tackle two significant business challenges: fraud detection and construction cost management. This case study explores these initiatives, detailing the implementation strategies, outcomes and broader implications for businesses.
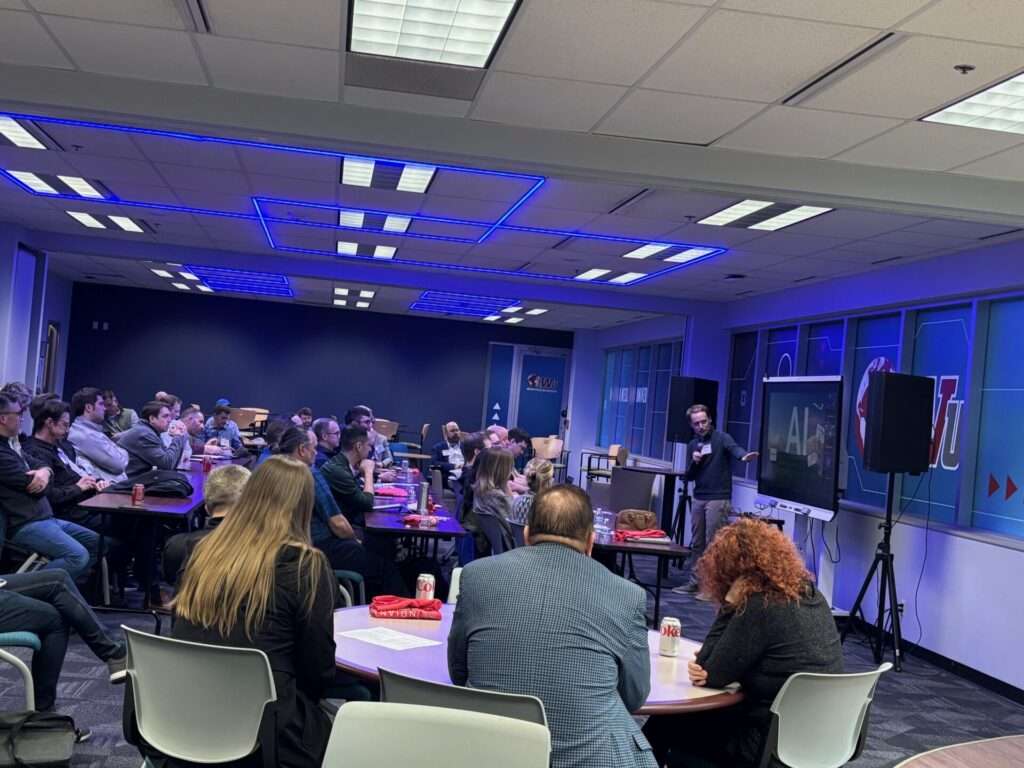
Part 1: Why Constraints Matter
AI is both a powerful tool and a broad concept. It is often presented as an all-encompassing solution, and the language surrounding AI varies widely. Some organizations offer their teams direct access to tools like ChatGPT, while others build AI for life-saving drug development. This variance of use makes it difficult for businesses to evaluate AI investments and determine how to use AI effectively.
Data317 has worked across industries, from startups that are building AI use-cases for the first time to multi-billion-dollar organizations that are using the tool to find ways to streamline operations. Regardless of whether the project is new, well-established, huge or small, one thing is clear: AI is only as successful as the constraints placed on it.
When companies launch AI projects, they typically focus on two elements:
- Objectives—the overarching goal, such as fraud detection, cost optimization or efficiency improvements.
- Setups—the supporting systems and workflows, including architecture and deployment strategies.
The most overlooked, yet critical factor for AI success, is constraints, or boundaries that define the problem and guide AI toward practical solutions.
AI projects fail when they try to solve problems that are too broad. Constraints help define the problem effectively, making solutions more actionable, efficient and scalable. The following case studies showcase how constraints played a pivotal role in ensuring successful AI implementations.
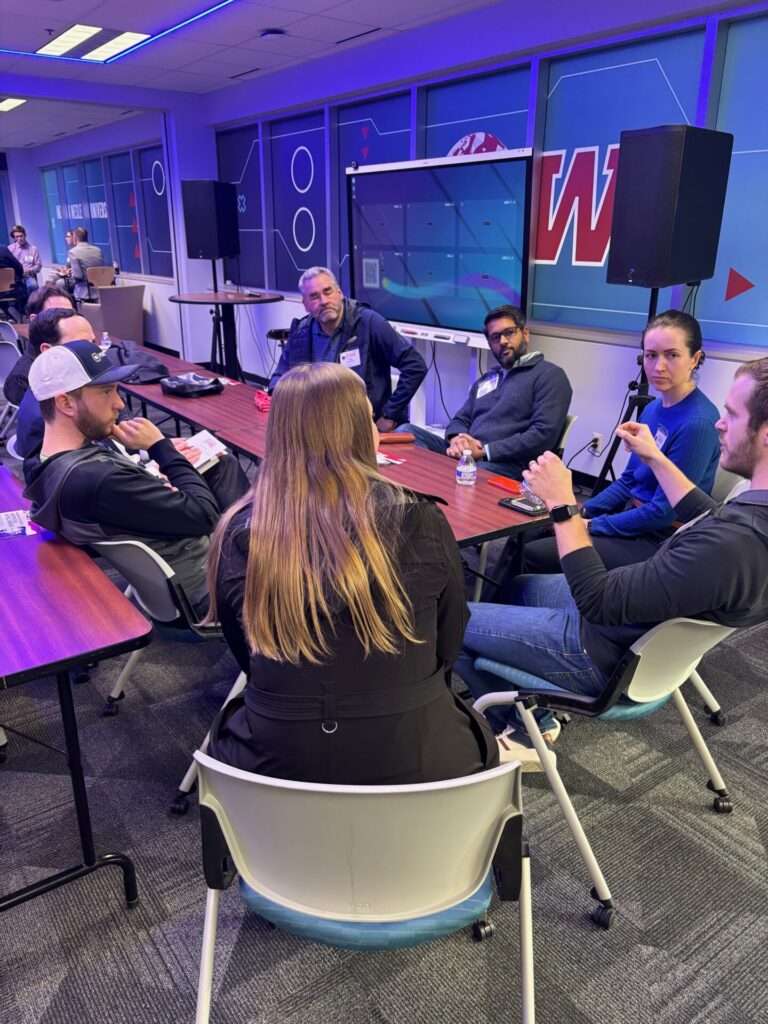
Part 2: AI-Driven Fraud Detection
The Challenge
A large organization faced a growing volume of fraudulent claims. Its traditional, rule-based system wasn’t scaling, and it took months for the system to detect fraud which led to substantial financial losses. With millions on the line, the company wanted to use AI for near real-time fraud detection.
Constraining the Problem
Initially, the problem was an open-ended effort to catch all forms of fraud. The natural instinct in such cases is to apply AI broadly– enhancing existing rule-based systems or training a deep learning model. However, these approaches are very expensive, opaque, and would require regular updates while also potentially generating unpredictable results, commonly known as hallucinations.
Data317 worked with the organization to narrow its focus and define clear constraints.
To build constraints, one must ask fundamental, sometimes obvious, questions. In this case, the key question was: What is fraud, really? For the organization, fraud was people making up fake transactions. Instead of attempting to identify every possible loophole and scheme, Data317 focused on detecting made-up numbers.
Solution & Implementation
By leveraging Benford’s Law, a mathematical principle that predicts the frequency of leading digits in naturally occurring datasets, the AI model was designed to detect numerical anomalies in the organization’s claims.
- Data Integration: The AI system seamlessly integrated with tax records for real-time anomaly detection.
- Model Training: Using historical tax data, the AI learned expected numerical distributions.
- Real-Time Detection: The system flagged potential fraud in minutes, compared to the months-long manual process.
Outcomes
- Millions in Fraud Detected: The system identified millions of fraudulent claims with a high degree of accuracy.
- Massive Time Savings: The time to detect fraud was reduced from months to mere moments.
- Better Resource Allocation: Investigators could focus on high-likelihood fraud cases, improving efficiency.
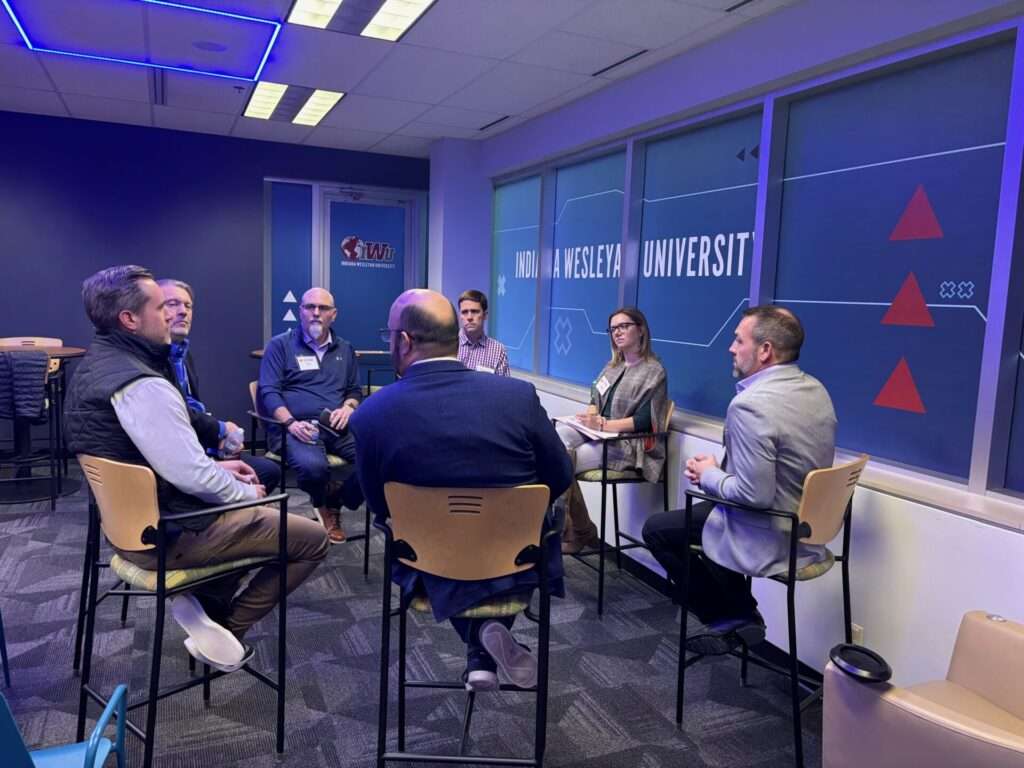
Part 3: Controlling Spiraling Construction Costs
The Challenge
During a period of significant supply chain disruptions and price volatility, a major construction firm faced escalating material costs, leading to cash flow uncertainties and overall business operation disruption. The primary challenge was the lack of a reliable method to differentiate between normal price fluctuations and potential internal errors, which often led to costly misjudgments.
Constraining the Problem
At first, the company considered using AI to predict pricing trends with time-series analyses. But the underlying data was inconsistent and not standardized, making accurate forecasting nearly impossible. Another approach was to use AI to correct material orders. But because millions of dollars flowed through the company’s system, hallucinations and untraceable corrections were unacceptable risks.
Instead of jumping to a complex AI solution, Data317 worked to narrow the problem by asking fundamental, almost obvious, questions:
- Why can’t we track material costs? Because the company couldn’t reliably link purchases to their actual costs.
- Why are there so many errors? Because material orders were hand-entered across multiple systems, leading to mismatched records and inconsistencies.
These questions reframed the problem. The company didn’t only need better forecasts or automated corrections—it needed AI to bring structure and traceability to its cost data. By focusing AI on linking, matching and validating transactions, the company could regain control over spending and eliminate the uncertainty that was making cost management so difficult. Once that foundation was in place, more advanced AI applications could actually work.
Solution & Implementation
With a constrained problem, Data317 implemented an AI-driven system to bring structure to cost tracking and management:
- Automated Data Matching: AI linked material orders to actual purchases, cleaning up inconsistencies across different systems.
- Cost Consolidation: The AI grouped similar purchases, standardizing item tracking and removing duplicate entries.
- Real-Time Anomaly Detection: The system flagged errors and unexpected cost spikes, allowing teams to intervene before they became major budget problems.
Outcomes
- Improved Cost Stability: By ensuring real-time tracking and validation, cost fluctuations became manageable rather than disruptive.
- Millions in Tracked Project Costs: With AI bringing structure to financial data, the company significantly improved budget forecasting and cash flow management.
- Increased Operational Flexibility: With greater confidence in their financial data, business leaders were able to allocate resources more effectively, reinvesting in high-priority projects rather than reacting to pricing uncertainties.
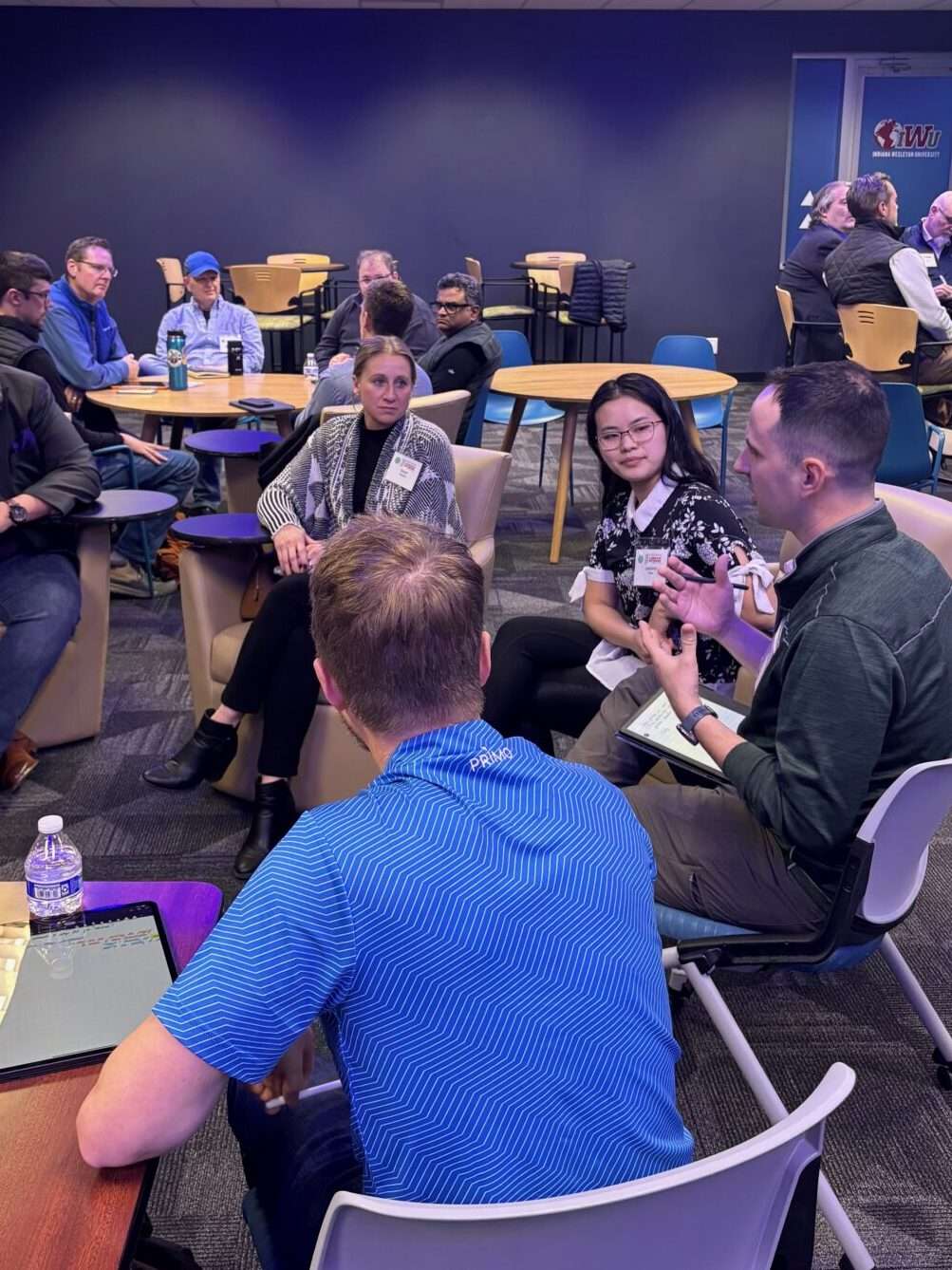
Part 4: Conclusion
After hearing the case study at the recent AI Innovation Network meeting, the network members broke into small groups to discuss and reflect. Key learnings highlighted common themes:
- As organizations continue to adopt AI, establishing a strong data foundation is critical. A crawl, walk, run approach allows teams to improve data quality while building AI capabilities.
- There is significant value in applying constraints to general prompts in generative AI tools such as ChatGPT/Perplexity.
- AI is only as good as the constraints placed on it. Defining the problem space is crucial for successful AI implementation.
Through these two examples, the Data317 team has demonstrated the importance of setting strong constraints when using AI to transform key business operations. These case studies serve as exemplars for implementing AI to solve complex challenges, providing valuable insights for other organizations aiming to leverage technology for strategic advantage.